Artificial intelligence (AI) has the potential to significantly transform India by addressing challenges in governance, healthcare, agriculture, financial services, and beyond. However, fully harnessing this potential requires designing AI systems tailored to India's unique linguistic, cultural, and infrastructural landscape. Achieving this necessitates the development of localized AI models, ensuring robust data sovereignty, and adopting a distributed approach to computing infrastructure.
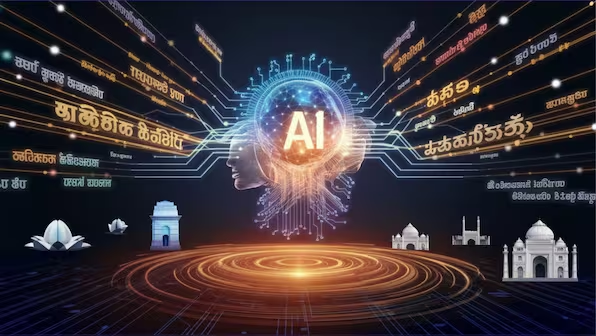
India’s strong government support, vast talent base, and rich data sources make it a country poised to reap tremendous benefits from AI. AI generated Image
The Importance of Localized AI Models
The most widely used AI models today are primarily trained on data in the English language. As a result, these models often reflect an Anglo-centric perspective from both linguistic and cultural standpoints. Given India's linguistic diversity, with 22 official languages and hundreds, if not thousands, of dialects, developing localized language models—whether large or small—becomes crucial for fostering inclusive AI adoption.
On one hand, it is essential to develop large language models (LLMs) that are trained on Indian language data. These models need to be tailored to capture the unique cultural nuances of India and generate text in Indian languages. Such localized AI models help minimize cultural biases and enhance the relevance of applications in areas like government services and education. They ensure that AI solutions are more inclusive and accessible to all Indians, including those who do not speak English. For example, the Indus LLM by Tech Mahindra is an indigenous foundational model designed to communicate in multiple Indic languages and dialects, implemented through an innovative "GenAI-in-a-box" framework.
On the other hand, small language models (SLMs), which are based on fewer parameters, can be more easily fine-tuned for specific localized tasks such as regional language translation or industry-specific customer service chatbots. These models also require significantly less computational power, making them ideal for environments that rely on AI-powered PCs, mobile devices, or edge computing. This allows AI to be deployed at scale, delivering societal benefits similar to the impact of Aadhaar and UPI.
To develop these models, vast datasets in Indian languages must be collected, which can be achieved through crowdsourcing and collaboration between academia, industry, and government. Moreover, while model development is crucial, it’s equally important to focus on applying these models to solve real-world challenges at scale, as this may be the key to driving widespread impact.
Data Localization: A Key Strategic Priority
India accounts for around 20 percent of the world’s data, yet much of it is stored on international servers. As data is crucial for AI, and we have an abundance of it, the need for data localization goes beyond security, compliance, and governance. It also holds substantial benefits for AI deployment.
Firstly, storing and processing data locally reduces latency from cross-border data transfers, leading to more efficient AI operations. Additionally, leveraging domestic data centers can be more cost-effective than using international cloud services, as subscription fees and cross-border data transfers are expensive. Local data also ensures better understanding of the local context, making the applications we develop more relevant.
Although the initial investment in setting up local data centers may be high, the Indian government and private sector are heavily investing in expanding data center capacity. This will ultimately reduce costs as economies of scale kick in and competition grows among local providers.
Moreover, supporting domestic data centers boosts the local economy by creating jobs and promoting technological progress. This fosters a stronger ecosystem for AI development, further lowering costs through collaboration and innovation.
Investing in local data centers, much like infrastructure development such as highways, is vital for India’s future as it strives to become an AI leader.
Adopting Distributed Computing for Enhanced Scalability
While the expansion of data centers in India is essential, the future of AI lies in distributed computing—utilizing a combination of centralized facilities, AI PCs, and edge computing.
There are several reasons for this. Firstly, relying solely on data centers or the cloud for AI can be costly. Whether it's owning, leasing, or subscribing to local cloud services, managing these operations can be complex and beyond the reach of many organizations. Secondly, although using domestic data centers is an improvement over relying on international ones, transmitting data back and forth between the source and the data centers can still cause delays, which is problematic for time-sensitive applications like payment systems and healthcare monitoring. Thirdly, even with data centers located within India, some organizations may prefer to store their data on-site for security and privacy reasons. Beyond issues of cost, latency, and security, there are also environmental concerns—such as India’s limited water resources—which make building large-scale centralized data centers challenging. We need to rethink how data centers are constructed, and a distributed computing model could provide a solution.
Computing must be decentralized across various locations and devices, utilizing different computing resources for various AI applications. With millions of PCs, edge devices, and around 950,000 cell towers, each point of computation can act as an AI engine. As computing power increases, there’s little need to run smaller language models or AI agents in a centralized data center when they can operate directly on a PC or at the edge. Edge computing allows for real-time processing, reduces network congestion, and can continue processing data even without internet connectivity—so why rely solely on data centers?
AI is inherently complex, with varying computing needs for different applications. To ensure AI accessibility for all, we must rethink infrastructure to meet India’s growing demand for computing resources.
Envisioning India's AI Future
According to the IDC Asia/Pacific AI Maturity Study 2024, commissioned by Intel, India is currently at stage 2 of AI maturity, positioned at the AI practitioner level. However, to advance in AI maturity, India needs to address the areas outlined earlier.
With strong government support, a vast talent pool, and abundant data resources, India is well-positioned to unlock significant benefits from AI. By prioritizing these key areas, India can harness the immense potential of AI to serve its citizens more equitably.
India is rapidly expanding its AI infrastructure, and it is crucial to ensure that the foundation being established today will serve the nation in the long run, with accessibility, sustainability, and inclusivity at its core.
Comments